Compressive SAR imaging with joint sparsity and local similarity exploitation
- PMID: 25686307
- PMCID: PMC4367404
- DOI: 10.3390/s150204176
Compressive SAR imaging with joint sparsity and local similarity exploitation
Abstract
Compressive sensing-based synthetic aperture radar (SAR) imaging has shown its superior capability in high-resolution image formation. However, most of those works focus on the scenes that can be sparsely represented in fixed spaces. When dealing with complicated scenes, these fixed spaces lack adaptivity in characterizing varied image contents. To solve this problem, a new compressive sensing-based radar imaging approach with adaptive sparse representation is proposed. Specifically, an autoregressive model is introduced to adaptively exploit the structural sparsity of an image. In addition, similarity among pixels is integrated into the autoregressive model to further promote the capability and thus an adaptive sparse representation facilitated by a weighted autoregressive model is derived. Since the weighted autoregressive model is inherently determined by the unknown image, we propose a joint optimization scheme by iterative SAR imaging and updating of the weighted autoregressive model to solve this problem. Eventually, experimental results demonstrated the validity and generality of the proposed approach.
Figures
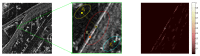
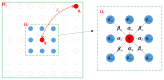
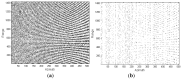
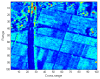
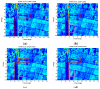
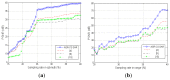
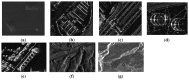
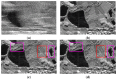
Similar articles
-
A sparsity-driven approach for joint SAR imaging and phase error correction.IEEE Trans Image Process. 2012 Apr;21(4):2075-88. doi: 10.1109/TIP.2011.2179056. Epub 2011 Dec 9. IEEE Trans Image Process. 2012. PMID: 22167627
-
Image deblurring and super-resolution by adaptive sparse domain selection and adaptive regularization.IEEE Trans Image Process. 2011 Jul;20(7):1838-57. doi: 10.1109/TIP.2011.2108306. Epub 2011 Jan 28. IEEE Trans Image Process. 2011. PMID: 21278019
-
Efficient Parameter Estimation for Sparse SAR Imaging Based on Complex Image and Azimuth-Range Decouple.Sensors (Basel). 2019 Oct 19;19(20):4549. doi: 10.3390/s19204549. Sensors (Basel). 2019. PMID: 31635086 Free PMC article.
-
Compressive Sensing Image Restoration Using Adaptive Curvelet Thresholding and Nonlocal Sparse Regularization.IEEE Trans Image Process. 2016 Jul;25(7):3126-3140. doi: 10.1109/TIP.2016.2562563. Epub 2016 May 3. IEEE Trans Image Process. 2016. PMID: 27164591
-
Compressed Sensing Radar Imaging: Fundamentals, Challenges, and Advances.Sensors (Basel). 2019 Jul 13;19(14):3100. doi: 10.3390/s19143100. Sensors (Basel). 2019. PMID: 31337039 Free PMC article. Review.
Cited by
-
A Robust Reweighted L₁-Minimization Imaging Algorithm for Passive Millimeter Wave SAIR in Near Field.Sensors (Basel). 2015 Sep 25;15(10):24945-60. doi: 10.3390/s151024945. Sensors (Basel). 2015. PMID: 26404282 Free PMC article.
-
Compressed Sensing Techniques Applied to Ultrasonic Imaging of Cargo Containers.Sensors (Basel). 2017 Jan 15;17(1):162. doi: 10.3390/s17010162. Sensors (Basel). 2017. PMID: 28098841 Free PMC article.
-
Multichannel and Wide-Angle SAR Imaging Based on Compressed Sensing.Sensors (Basel). 2017 Feb 5;17(2):295. doi: 10.3390/s17020295. Sensors (Basel). 2017. PMID: 28165433 Free PMC article.
References
-
- Candès E., Wakin M.B. An introduction to compressive sampling. IEEE Signal Process. Mag. 2008;25:21–30.
-
- Candès E., Romberg J.K., Tao T. Stable signal recovery from incomplete and inaccurate measurements. Commun. Pure Appl. Math. 2006;59:1207–1223.
-
- Baraniuk R.G., Steeghs P. Compressive radar imaging. Proceedings of the IEEE Radar Conference; Boston, MA, USA. 17– 20 April 2007; pp. 128–133.
-
- Patel V.M., Easley G.R., Healy D.M., Jr., Chellappa R. Compressed synthetic aperture radar. IEEE J. Sel. Top. Signal Process. 2010;4:244–254.
-
- Tello Alonso M., Lopez-Dekker P., Mallorqui J. A novel strategy for radar imaging based on compressive sensing. IEEE Trans. Geosci. Remote Sens. 2010;48:4285–4295.
Publication types
MeSH terms
LinkOut - more resources
Full Text Sources
Other Literature Sources
Medical
Miscellaneous