Efficient lossy compression for compressive sensing acquisition of images in compressive sensing imaging systems
- PMID: 25490597
- PMCID: PMC4299070
- DOI: 10.3390/s141223398
Efficient lossy compression for compressive sensing acquisition of images in compressive sensing imaging systems
Abstract
Compressive Sensing Imaging (CSI) is a new framework for image acquisition, which enables the simultaneous acquisition and compression of a scene. Since the characteristics of Compressive Sensing (CS) acquisition are very different from traditional image acquisition, the general image compression solution may not work well. In this paper, we propose an efficient lossy compression solution for CS acquisition of images by considering the distinctive features of the CSI. First, we design an adaptive compressive sensing acquisition method for images according to the sampling rate, which could achieve better CS reconstruction quality for the acquired image. Second, we develop a universal quantization for the obtained CS measurements from CS acquisition without knowing any a priori information about the captured image. Finally, we apply these two methods in the CSI system for efficient lossy compression of CS acquisition. Simulation results demonstrate that the proposed solution improves the rate-distortion performance by 0.4~2 dB comparing with current state-of-the-art, while maintaining a low computational complexity.
Figures
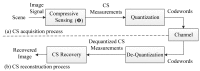
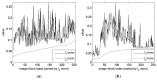
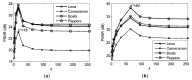
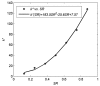
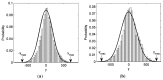
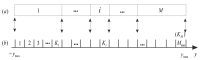
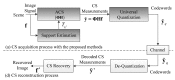
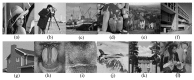
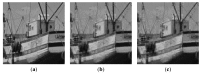
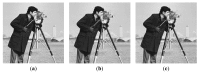
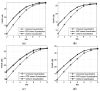
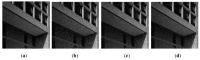
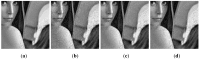

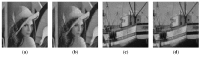
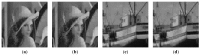
Similar articles
-
Low-Complexity Rate-Distortion Optimization of Sampling Rate and Bit-Depth for Compressed Sensing of Images.Entropy (Basel). 2020 Jan 20;22(1):125. doi: 10.3390/e22010125. Entropy (Basel). 2020. PMID: 33285900 Free PMC article.
-
Binned progressive quantization for compressive sensing.IEEE Trans Image Process. 2012 Jun;21(6):2980-90. doi: 10.1109/TIP.2012.2188810. Epub 2012 Feb 24. IEEE Trans Image Process. 2012. PMID: 22374362
-
Compressive sensing of sparse tensors.IEEE Trans Image Process. 2014 Oct;23(10):4438-47. doi: 10.1109/TIP.2014.2348796. Epub 2014 Aug 15. IEEE Trans Image Process. 2014. PMID: 25137727
-
Application of Compressive Sensing to Ultrasound Images: A Review.Biomed Res Int. 2019 Nov 15;2019:7861651. doi: 10.1155/2019/7861651. eCollection 2019. Biomed Res Int. 2019. PMID: 31828130 Free PMC article. Review.
-
Compressive Sensing Hyperspectral Imaging by Spectral Multiplexing with Liquid Crystal.J Imaging. 2018 Dec 22;5(1):3. doi: 10.3390/jimaging5010003. J Imaging. 2018. PMID: 34470182 Free PMC article. Review.
Cited by
-
Compressed Sensing Techniques Applied to Ultrasonic Imaging of Cargo Containers.Sensors (Basel). 2017 Jan 15;17(1):162. doi: 10.3390/s17010162. Sensors (Basel). 2017. PMID: 28098841 Free PMC article.
-
Low-Complexity Rate-Distortion Optimization of Sampling Rate and Bit-Depth for Compressed Sensing of Images.Entropy (Basel). 2020 Jan 20;22(1):125. doi: 10.3390/e22010125. Entropy (Basel). 2020. PMID: 33285900 Free PMC article.
-
A General Rate-Distortion Optimization Method for Block Compressed Sensing of Images.Entropy (Basel). 2021 Oct 16;23(10):1354. doi: 10.3390/e23101354. Entropy (Basel). 2021. PMID: 34682078 Free PMC article.
-
A Convolutional Neural Network-Based Quantization Method for Block Compressed Sensing of Images.Entropy (Basel). 2024 May 29;26(6):468. doi: 10.3390/e26060468. Entropy (Basel). 2024. PMID: 38920476 Free PMC article.
References
-
- Unser M. Sampling-50 years after Shannon. Proc. IEEE. 2000;88:569–587.
-
- Li C., Jiang H., Wilford P., Zhang Y., Scheutzow M. A new compressive video sensing framework for mobile broadcast. IEEE Trans. Broadcast. 2013;59:197–205.
-
- Akyildiz I.F., Melodia T., Chowdhury K.R. A survey on wireless multimedia sensor networks. Comput. Netw. 2007;51:921–960.
-
- Pudlewski S., Melodia T., Prasanna A. Compressed-sensing enabled video streaming for wireless multimedia sensor networks. IEEE Trans. Mob. Comput. 2012;11:1060–1072.
-
- Blanes I., Magli E., Serra-Sagrista J. A tutorial on image compression for optical space imaging systems. IEEE Trans. Geosci. Remote Sens. Mag. 2014;2:8–26.
LinkOut - more resources
Full Text Sources
Other Literature Sources
Miscellaneous