Nonuniform sampling and non-Fourier signal processing methods in multidimensional NMR
- PMID: 25456315
- PMCID: PMC5776146
- DOI: 10.1016/j.pnmrs.2014.09.002
Nonuniform sampling and non-Fourier signal processing methods in multidimensional NMR
Erratum in
- Prog Nucl Magn Reson Spectrosc. 2015 Apr;86-87;80
Abstract
Beginning with the introduction of Fourier Transform NMR by Ernst and Anderson in 1966, time domain measurement of the impulse response (the free induction decay, FID) consisted of sampling the signal at a series of discrete intervals. For compatibility with the discrete Fourier transform (DFT), the intervals are kept uniform, and the Nyquist theorem dictates the largest value of the interval sufficient to avoid aliasing. With the proposal by Jeener of parametric sampling along an indirect time dimension, extension to multidimensional experiments employed the same sampling techniques used in one dimension, similarly subject to the Nyquist condition and suitable for processing via the discrete Fourier transform. The challenges of obtaining high-resolution spectral estimates from short data records using the DFT were already well understood, however. Despite techniques such as linear prediction extrapolation, the achievable resolution in the indirect dimensions is limited by practical constraints on measuring time. The advent of non-Fourier methods of spectrum analysis capable of processing nonuniformly sampled data has led to an explosion in the development of novel sampling strategies that avoid the limits on resolution and measurement time imposed by uniform sampling. The first part of this review discusses the many approaches to data sampling in multidimensional NMR, the second part highlights commonly used methods for signal processing of such data, and the review concludes with a discussion of other approaches to speeding up data acquisition in NMR.
Keywords: Fast acquisition; Multidimensional NMR; Non-uniform sampling; Reduced dimensionality; Signal processing.
Copyright © 2014 Elsevier B.V. All rights reserved.
Figures
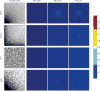
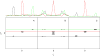
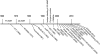
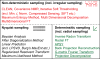
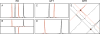
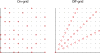
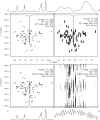
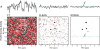
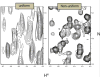
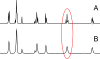
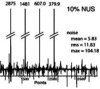
Similar articles
-
Data sampling in multidimensional NMR: fundamentals and strategies.Top Curr Chem. 2012;316:49-77. doi: 10.1007/128_2011_185. Top Curr Chem. 2012. PMID: 21773916 Free PMC article. Review.
-
Nonuniform sampling and maximum entropy reconstruction in multidimensional NMR.Acc Chem Res. 2014 Feb 18;47(2):708-17. doi: 10.1021/ar400244v. Epub 2014 Jan 9. Acc Chem Res. 2014. PMID: 24400700 Free PMC article.
-
Beyond Fourier.J Magn Reson. 2017 Oct;283:117-123. doi: 10.1016/j.jmr.2017.03.017. Epub 2017 Mar 27. J Magn Reson. 2017. PMID: 28385517 Free PMC article.
-
Nonuniform sampling of hypercomplex multidimensional NMR experiments: Dimensionality, quadrature phase and randomization.J Magn Reson. 2015 May;254:121-30. doi: 10.1016/j.jmr.2015.02.015. Epub 2015 Mar 10. J Magn Reson. 2015. PMID: 25899289 Free PMC article.
-
Generalized Fourier transform for non-uniform sampled data.Top Curr Chem. 2012;316:79-124. doi: 10.1007/128_2011_186. Top Curr Chem. 2012. PMID: 21769718 Review.
Cited by
-
Accurate determination of rates from non-uniformly sampled relaxation data.J Biomol NMR. 2016 Aug;65(3-4):157-170. doi: 10.1007/s10858-016-0046-9. Epub 2016 Jul 8. J Biomol NMR. 2016. PMID: 27393626 Free PMC article.
-
Nonuniform sampling in multidimensional NMR for improving spectral sensitivity.Methods. 2018 Apr 1;138-139:62-68. doi: 10.1016/j.ymeth.2018.03.001. Epub 2018 Mar 6. Methods. 2018. PMID: 29522805 Free PMC article.
-
Characterization of backbone dynamics using solution NMR spectroscopy to discern the functional plasticity of structurally analogous proteins.STAR Protoc. 2021 Oct 29;2(4):100919. doi: 10.1016/j.xpro.2021.100919. eCollection 2021 Dec 17. STAR Protoc. 2021. PMID: 34761231 Free PMC article.
-
Accelerating 2D NMR relaxation dispersion experiments using iterated maps.J Biomol NMR. 2019 Nov;73(10-11):561-576. doi: 10.1007/s10858-019-00263-3. Epub 2019 Jul 6. J Biomol NMR. 2019. PMID: 31280454 Free PMC article.
-
Structural basis for the binding of the cancer targeting scorpion toxin, ClTx, to the vascular endothelia growth factor receptor neuropilin-1.Curr Res Struct Biol. 2021 Aug 2;3:179-186. doi: 10.1016/j.crstbi.2021.07.003. eCollection 2021. Curr Res Struct Biol. 2021. PMID: 34401749 Free PMC article.
References
-
- Ernst RR, Anderson WA. Rev Sci Instrum. 1966;37:93.
-
- Hoch JC, Stern AS. NMR Data Processing. Wiley-Liss; New York: 1996.
-
- Beckman RA, Zuiderweg ERP. J Magn Reson. 1995;A113:223.
-
- Delsuc MA, Lallemand JY. J Magn Reson. 1986;69:504.
-
- Rovnyak D, Hoch JC, Stern AS, Wagner G. J Biomol NMR. 2004;30:1. - PubMed
Publication types
MeSH terms
Grants and funding
LinkOut - more resources
Full Text Sources
Other Literature Sources