Mutual information between discrete and continuous data sets
- PMID: 24586270
- PMCID: PMC3929353
- DOI: 10.1371/journal.pone.0087357
Mutual information between discrete and continuous data sets
Abstract
Mutual information (MI) is a powerful method for detecting relationships between data sets. There are accurate methods for estimating MI that avoid problems with "binning" when both data sets are discrete or when both data sets are continuous. We present an accurate, non-binning MI estimator for the case of one discrete data set and one continuous data set. This case applies when measuring, for example, the relationship between base sequence and gene expression level, or the effect of a cancer drug on patient survival time. We also show how our method can be adapted to calculate the Jensen-Shannon divergence of two or more data sets.
Conflict of interest statement
Figures
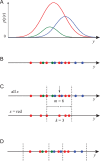




















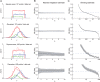








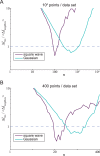






Similar articles
-
MIA: Mutual Information Analyzer, a graphic user interface program that calculates entropy, vertical and horizontal mutual information of molecular sequence sets.BMC Bioinformatics. 2015 Dec 10;16:409. doi: 10.1186/s12859-015-0837-0. BMC Bioinformatics. 2015. PMID: 26652707 Free PMC article.
-
[Comparison study on the methods for finding borders between coding and non-coding DNA regions in rice].Yi Chuan. 2005 Jul;27(4):629-35. Yi Chuan. 2005. PMID: 16120591 Chinese.
-
Approximations of Shannon Mutual Information for Discrete Variables with Applications to Neural Population Coding.Entropy (Basel). 2019 Mar 4;21(3):243. doi: 10.3390/e21030243. Entropy (Basel). 2019. PMID: 33266958 Free PMC article.
-
Discrete dynamic modeling with asynchronous update, or how to model complex systems in the absence of quantitative information.Methods Mol Biol. 2009;553:207-25. doi: 10.1007/978-1-60327-563-7_10. Methods Mol Biol. 2009. PMID: 19588107 Review.
-
Genes, information and sense: complexity and knowledge retrieval.Theory Biosci. 2008 Jun;127(2):69-78. doi: 10.1007/s12064-008-0032-1. Epub 2008 Apr 29. Theory Biosci. 2008. PMID: 18443840 Review.
Cited by
-
Automated CT Lung Density Analysis of Viral Pneumonia and Healthy Lungs Using Deep Learning-Based Segmentation, Histograms and HU Thresholds.Diagnostics (Basel). 2021 Apr 21;11(5):738. doi: 10.3390/diagnostics11050738. Diagnostics (Basel). 2021. PMID: 33919094 Free PMC article.
-
Cell type-specific genome scans of DNA methylation divergence indicate an important role for transposable elements.Genome Biol. 2020 Jul 13;21(1):172. doi: 10.1186/s13059-020-02068-2. Genome Biol. 2020. PMID: 32660534 Free PMC article.
-
Perceived Realism of High-Resolution Generative Adversarial Network-derived Synthetic Mammograms.Radiol Artif Intell. 2020 Dec 23;3(2):e190181. doi: 10.1148/ryai.2020190181. eCollection 2021 Mar. Radiol Artif Intell. 2020. PMID: 33937856 Free PMC article.
-
Estimating Prevalence and Characteristics of Statin Intolerance among High and Very High Cardiovascular Risk Patients in Germany (2017 to 2020).J Clin Med. 2023 Jan 16;12(2):705. doi: 10.3390/jcm12020705. J Clin Med. 2023. PMID: 36675634 Free PMC article.
-
Epidemiology of rabies immune globulin use in paediatric and adult patients in the USA: a cross-sectional prevalence study.BMJ Open. 2022 Apr 26;12(4):e055411. doi: 10.1136/bmjopen-2021-055411. BMJ Open. 2022. PMID: 35473745 Free PMC article.
References
-
- Cover T, Thomas J (1991) Elements of information theory. New York: John Wiley & Sons.
-
- Kraskov A, Stögbauer H, Grassberger P (2004) Estimating mutual information. Physical Review E 69: 066138. - PubMed
-
- Grosse I, Bernaola-Galván P, Carpena P, Román-Roldán R, Oliver J, et al. (2002) Analysis of symbolic sequences using the jensen-shannon divergence. Physical Review E 65: 041905. - PubMed
-
- Abramowitz M, Stegun I (1970) Handbook of mathematical functions. New York: Dover Publishing Inc.
-
- Kozachenko L, Leonenko NN (1987) Sample estimate of the entropy of a random vector. Problemy Peredachi Informatsii 23: 9–16.
MeSH terms
Substances
Grants and funding
LinkOut - more resources
Full Text Sources
Other Literature Sources