Learning what to expect (in visual perception)
- PMID: 24187536
- PMCID: PMC3807544
- DOI: 10.3389/fnhum.2013.00668
Learning what to expect (in visual perception)
Abstract
Expectations are known to greatly affect our experience of the world. A growing theory in computational neuroscience is that perception can be successfully described using Bayesian inference models and that the brain is "Bayes-optimal" under some constraints. In this context, expectations are particularly interesting, because they can be viewed as prior beliefs in the statistical inference process. A number of questions remain unsolved, however, for example: How fast do priors change over time? Are there limits in the complexity of the priors that can be learned? How do an individual's priors compare to the true scene statistics? Can we unlearn priors that are thought to correspond to natural scene statistics? Where and what are the neural substrate of priors? Focusing on the perception of visual motion, we here review recent studies from our laboratories and others addressing these issues. We discuss how these data on motion perception fit within the broader literature on perceptual Bayesian priors, perceptual expectations, and statistical and perceptual learning and review the possible neural basis of priors.
Keywords: Bayesian priors; expectations; perceptual learning; probabilistic inference; statistical learning.
Figures
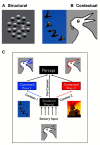
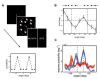
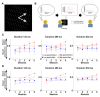
Similar articles
-
Learning What to See in a Changing World.Front Hum Neurosci. 2016 May 31;10:263. doi: 10.3389/fnhum.2016.00263. eCollection 2016. Front Hum Neurosci. 2016. PMID: 27303285 Free PMC article.
-
Acquisition of visual priors and induced hallucinations in chronic schizophrenia.Brain. 2019 Aug 1;142(8):2523-2537. doi: 10.1093/brain/awz171. Brain. 2019. PMID: 31257444 Free PMC article.
-
Complexity and specificity of experimentally-induced expectations in motion perception.J Vis. 2013 Mar 13;13(4):8. doi: 10.1167/13.4.8. J Vis. 2013. PMID: 23487160
-
Priors in perception: Top-down modulation, Bayesian perceptual learning rate, and prediction error minimization.Conscious Cogn. 2017 Jan;47:75-85. doi: 10.1016/j.concog.2016.09.004. Epub 2016 Sep 20. Conscious Cogn. 2017. PMID: 27663763 Review.
-
Computational Neuropsychology and Bayesian Inference.Front Hum Neurosci. 2018 Feb 23;12:61. doi: 10.3389/fnhum.2018.00061. eCollection 2018. Front Hum Neurosci. 2018. PMID: 29527157 Free PMC article. Review.
Cited by
-
Predictions Shape Confidence in Right Inferior Frontal Gyrus.J Neurosci. 2016 Oct 5;36(40):10323-10336. doi: 10.1523/JNEUROSCI.1092-16.2016. J Neurosci. 2016. PMID: 27707969 Free PMC article.
-
Suboptimality in perceptual decision making.Behav Brain Sci. 2018 Feb 27;41:e223. doi: 10.1017/S0140525X18000936. Behav Brain Sci. 2018. PMID: 29485020 Free PMC article.
-
Sensory and environmental uncertainty in perceptual decision-making.iScience. 2023 Mar 15;26(4):106412. doi: 10.1016/j.isci.2023.106412. eCollection 2023 Apr 21. iScience. 2023. PMID: 37035003 Free PMC article.
-
Recent insights into perceptual and motor skill learning.Front Hum Neurosci. 2014 Sep 3;8:683. doi: 10.3389/fnhum.2014.00683. eCollection 2014. Front Hum Neurosci. 2014. PMID: 25232311 Free PMC article. No abstract available.
-
The basal ganglia select the expected sensory input used for predictive coding.Front Comput Neurosci. 2015 Sep 23;9:119. doi: 10.3389/fncom.2015.00119. eCollection 2015. Front Comput Neurosci. 2015. PMID: 26441627 Free PMC article.
References
Publication types
LinkOut - more resources
Full Text Sources
Other Literature Sources