Mechanisms for stable, robust, and adaptive development of orientation maps in the primary visual cortex
- PMID: 24089483
- PMCID: PMC6618482
- DOI: 10.1523/JNEUROSCI.1037-13.2013
Mechanisms for stable, robust, and adaptive development of orientation maps in the primary visual cortex
Abstract
Development of orientation maps in ferret and cat primary visual cortex (V1) has been shown to be stable, in that the earliest measurable maps are similar in form to the eventual adult map, robust, in that similar maps develop in both dark rearing and in a variety of normal visual environments, and yet adaptive, in that the final map pattern reflects the statistics of the specific visual environment. How can these three properties be reconciled? Using mechanistic models of the development of neural connectivity in V1, we show for the first time that realistic stable, robust, and adaptive map development can be achieved by including two low-level mechanisms originally motivated from single-neuron results. Specifically, contrast-gain control in the retinal ganglion cells and the lateral geniculate nucleus reduces variation in the presynaptic drive due to differences in input patterns, while homeostatic plasticity of V1 neuron excitability reduces the postsynaptic variability in firing rates. Together these two mechanisms, thought to be applicable across sensory systems in general, lead to biological maps that develop stably and robustly, yet adapt to the visual environment. The modeling results suggest that topographic map stability is a natural outcome of low-level processes of adaptation and normalization. The resulting model is more realistic, simpler, and far more robust, and is thus a good starting point for future studies of cortical map development.
Figures

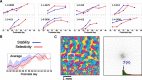
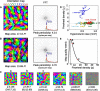
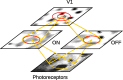
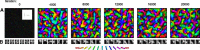
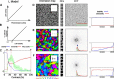
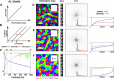
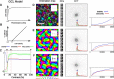
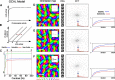
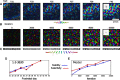
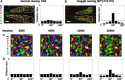
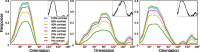
Similar articles
-
Interlayer Repulsion of Retinal Ganglion Cell Mosaics Regulates Spatial Organization of Functional Maps in the Visual Cortex.J Neurosci. 2017 Dec 13;37(50):12141-12152. doi: 10.1523/JNEUROSCI.1873-17.2017. Epub 2017 Nov 7. J Neurosci. 2017. PMID: 29114075 Free PMC article.
-
Color opponent receptive fields self-organize in a biophysical model of visual cortex via spike-timing dependent plasticity.Front Neural Circuits. 2014 Mar 12;8:16. doi: 10.3389/fncir.2014.00016. eCollection 2014. Front Neural Circuits. 2014. PMID: 24659956 Free PMC article.
-
Orientation Selectivity from Very Sparse LGN Inputs in a Comprehensive Model of Macaque V1 Cortex.J Neurosci. 2016 Dec 7;36(49):12368-12384. doi: 10.1523/JNEUROSCI.2603-16.2016. J Neurosci. 2016. PMID: 27927956 Free PMC article.
-
Functional cell classes and functional architecture in the early visual system of a highly visual rodent.Prog Brain Res. 2005;149:127-45. doi: 10.1016/S0079-6123(05)49010-X. Prog Brain Res. 2005. PMID: 16226581 Review.
-
Origins of feature selectivities and maps in the mammalian primary visual cortex.Trends Neurosci. 2015 Aug;38(8):475-85. doi: 10.1016/j.tins.2015.06.003. Epub 2015 Jul 22. Trends Neurosci. 2015. PMID: 26209463 Review.
Cited by
-
Analytic Model for Feature Maps in the Primary Visual Cortex.Front Comput Neurosci. 2022 Feb 4;16:659316. doi: 10.3389/fncom.2022.659316. eCollection 2022. Front Comput Neurosci. 2022. PMID: 35185503 Free PMC article.
-
Stable memory and computation in randomly rewiring neural networks.J Neurophysiol. 2019 Jul 1;122(1):66-80. doi: 10.1152/jn.00534.2018. Epub 2019 Apr 10. J Neurophysiol. 2019. PMID: 30969897 Free PMC article.
-
Computational Modeling of Contrast Sensitivity and Orientation Tuning in First-Episode and Chronic Schizophrenia.Comput Psychiatr. 2017 Dec 1;1:102-131. doi: 10.1162/CPSY_a_00005. eCollection 2017 Dec. Comput Psychiatr. 2017. PMID: 30090855 Free PMC article.
-
An automated and reproducible workflow for running and analyzing neural simulations using Lancet and IPython Notebook.Front Neuroinform. 2013 Dec 30;7:44. doi: 10.3389/fninf.2013.00044. eCollection 2013. Front Neuroinform. 2013. PMID: 24416014 Free PMC article.
-
Improving voltage-sensitive dye imaging: with a little help from computational approaches.Neurophotonics. 2017 Jul;4(3):031215. doi: 10.1117/1.NPh.4.3.031215. Epub 2017 May 19. Neurophotonics. 2017. PMID: 28573154 Free PMC article.
References
-
- Anderson JS, Carandini M, Ferster D. Orientation tuning of input conductance, excitation, and inhibition in cat primary visual cortex. J Neurophysiol. 2000;84:909–926. - PubMed
-
- Antolík J. Edinburgh, UK: School of Informatics, The University of Edinburgh; 2010. Unified developmental model of maps, complex cells and surround modulation in the primary visual cortex. PhD thesis.
Publication types
MeSH terms
Grants and funding
LinkOut - more resources
Full Text Sources
Other Literature Sources
Molecular Biology Databases
Miscellaneous