fMRI-DTI modeling via landmark distance atlases for prediction and detection of fiber tracts
- PMID: 22155376
- PMCID: PMC3423975
- DOI: 10.1016/j.neuroimage.2011.11.014
fMRI-DTI modeling via landmark distance atlases for prediction and detection of fiber tracts
Abstract
The overall goal of this research is the design of statistical atlas models that can be created from normal subjects, but may generalize to be applicable to abnormal brains. We present a new style of joint modeling of fMRI, DTI, and structural MRI. Motivated by the fact that a white matter tract and related cortical areas are likely to displace together in the presence of a mass lesion (brain tumor), in this work we propose a rotation and translation invariant model that represents the spatial relationship between fiber tracts and anatomic and functional landmarks. This landmark distance model provides a new basis for representation of fiber tracts and can be used for detection and prediction of fiber tracts based on landmarks. Our results indicate that the measured model is consistent across normal subjects, and thus suitable for atlas building. Our experiments demonstrate that the model is robust to displacement and missing data, and can be successfully applied to a small group of patients with mass lesions.
Copyright © 2011 Elsevier Inc. All rights reserved.
Figures
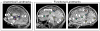
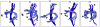
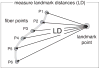
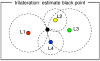
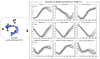
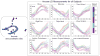
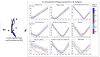
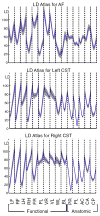
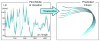
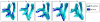
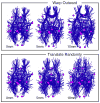
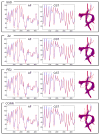
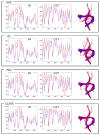
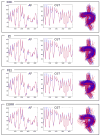
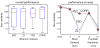
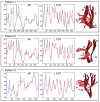
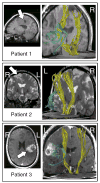
Similar articles
-
An anatomically curated fiber clustering white matter atlas for consistent white matter tract parcellation across the lifespan.Neuroimage. 2018 Oct 1;179:429-447. doi: 10.1016/j.neuroimage.2018.06.027. Epub 2018 Jun 18. Neuroimage. 2018. PMID: 29920375 Free PMC article.
-
Joint representation of consistent structural and functional profiles for identification of common cortical landmarks.Brain Imaging Behav. 2018 Jun;12(3):728-742. doi: 10.1007/s11682-017-9736-5. Brain Imaging Behav. 2018. PMID: 28597338 Free PMC article.
-
Associations between clinical outcome and navigated transcranial magnetic stimulation characteristics in patients with motor-eloquent brain lesions: a combined navigated transcranial magnetic stimulation-diffusion tensor imaging fiber tracking approach.J Neurosurg. 2018 Mar;128(3):800-810. doi: 10.3171/2016.11.JNS162322. Epub 2017 Mar 31. J Neurosurg. 2018. PMID: 28362239
-
[Quantitative white matter analysis by diffusion tensor imaging and potential functional correlation].Brain Nerve. 2011 Dec;63(12):1319-29. Brain Nerve. 2011. PMID: 22147451 Review. Japanese.
-
Diffusion tensor imaging: a review for pediatric researchers and clinicians.J Dev Behav Pediatr. 2010 May;31(4):346-56. doi: 10.1097/DBP.0b013e3181dcaa8b. J Dev Behav Pediatr. 2010. PMID: 20453582 Free PMC article. Review.
Cited by
-
An Example-Based Multi-Atlas Approach to Automatic Labeling of White Matter Tracts.PLoS One. 2015 Jul 30;10(7):e0133337. doi: 10.1371/journal.pone.0133337. eCollection 2015. PLoS One. 2015. PMID: 26225419 Free PMC article.
-
Automated multi-subject fiber clustering of mouse brain using dominant sets.Front Neuroinform. 2015 Jan 12;8:87. doi: 10.3389/fninf.2014.00087. eCollection 2014. Front Neuroinform. 2015. PMID: 25628561 Free PMC article.
-
Fusing DTI and fMRI data: a survey of methods and applications.Neuroimage. 2014 Nov 15;102 Pt 1:184-91. doi: 10.1016/j.neuroimage.2013.09.071. Epub 2013 Oct 5. Neuroimage. 2014. PMID: 24103849 Free PMC article. Review.
-
Unbiased groupwise registration of white matter tractography.Med Image Comput Comput Assist Interv. 2012;15(Pt 3):123-30. doi: 10.1007/978-3-642-33454-2_16. Med Image Comput Comput Assist Interv. 2012. PMID: 23286122 Free PMC article.
-
Personalised, image-guided, noninvasive brain stimulation in gliomas: Rationale, challenges and opportunities.EBioMedicine. 2021 Aug;70:103514. doi: 10.1016/j.ebiom.2021.103514. Epub 2021 Aug 11. EBioMedicine. 2021. PMID: 34391090 Free PMC article. Review.
References
-
- Catani M, de Schotten T. A diffusion tensor imaging tractography atlas for virtual in vivo dissections. Cortex. 2008;44(8):1105–1132. - PubMed
-
- Durrleman S, Fillard P, Pennec X, Trouvé A, Ayache N. A statistical model of white matter fiber bundles based on currents. In: Prince JL, Pham DL, Meyers KJ, editors. International Conference on Information Processing in Medical Imaging : Lecture Notes in Computer Science; Springer-Verlag; 2009. pp. 114–125. URL http://hal.inria.fr/inria-00502721/en/ - PubMed
-
- Fischl B, Salat D, Busa E, Albert M, Dieterich M, Haselgrove C, van der Kouwe A, Killiany R, Kennedy D, Klaveness S, et al. Whole brain segmentation: automated labeling of neuroanatomical structures in the human brain. Neuron. 2002;33(3):341–355. - PubMed
-
- Glasser MF, Rilling JK. DTI tractography of the human brain’s language pathways. Cereb Cortex. 2008;18(11):2471–2482. - PubMed
Publication types
MeSH terms
Grants and funding
- R25CA089017/CA/NCI NIH HHS/United States
- P41RR013218/RR/NCRR NIH HHS/United States
- P41RR019703/RR/NCRR NIH HHS/United States
- P41 RR019703/RR/NCRR NIH HHS/United States
- R21 CA156943/CA/NCI NIH HHS/United States
- P01CA067165/CA/NCI NIH HHS/United States
- R25 CA089017/CA/NCI NIH HHS/United States
- P41 RR013218/RR/NCRR NIH HHS/United States
- R01 MH074794/MH/NIMH NIH HHS/United States
- R01MH074794/MH/NIMH NIH HHS/United States
- R01 MH092862/MH/NIMH NIH HHS/United States
- 1R21CA156943-01A1/CA/NCI NIH HHS/United States
- P01 CA067165/CA/NCI NIH HHS/United States
- R01MH092862/MH/NIMH NIH HHS/United States
- P41 EB015902/EB/NIBIB NIH HHS/United States
LinkOut - more resources
Full Text Sources
Other Literature Sources
Medical