A unifying framework underlying mechanotransduction in the somatosensory system
- PMID: 21653856
- PMCID: PMC6623321
- DOI: 10.1523/JNEUROSCI.6695-10.2011
A unifying framework underlying mechanotransduction in the somatosensory system
Abstract
Rodents use their whiskers to sense their surroundings. As most of the information available to the somatosensory system originates in whiskers' primary afferents, it is essential to understand the transformation of whisker motion into neuronal activity. Here, we combined in vivo recordings in anesthetized rats with mathematical modeling to ascertain the mechanical and electrical characteristics of mechanotransduction. We found that only two synergistic processes, which reflect the dynamic interactions between (1) receptor and whisker and (2) receptor and surrounding tissue, are needed to describe mechanotransduction during passive whiskers deflection. Interactions between these processes may account for stimulus-dependent changes in the magnitude and temporal pattern of tactile responses on multiple scales. Thus, we are able to explain complex electromechanical processes underlying sensory transduction using a simple model, which captures the responses of a wide range of mechanoreceptor types to diverse sensory stimuli. This compact and precise model allows for a ubiquitous description of how mechanoreceptors encode tactile stimulus.
Figures
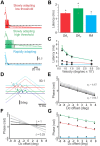
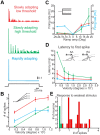
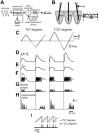
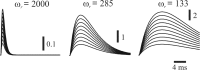
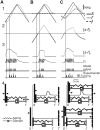
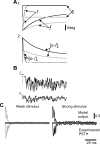
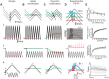
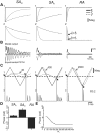
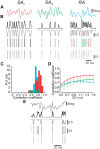
Similar articles
-
Texture coding in the rat whisker system: slip-stick versus differential resonance.PLoS Biol. 2008 Aug 26;6(8):e215. doi: 10.1371/journal.pbio.0060215. PLoS Biol. 2008. PMID: 18752354 Free PMC article.
-
Effects of neonatal C-fiber depletion on the integration of paired-whisker inputs in rat barrel cortex.Exp Brain Res. 2005 Mar;162(1):115-21. doi: 10.1007/s00221-004-2118-4. Epub 2004 Nov 13. Exp Brain Res. 2005. PMID: 15551079
-
Rate code and temporal code for frequency of whisker stimulation in rat primary and secondary somatic sensory cortex.Exp Brain Res. 2006 Jul;172(3):370-86. doi: 10.1007/s00221-005-0334-1. Epub 2006 Feb 3. Exp Brain Res. 2006. PMID: 16456683
-
Intracortical processes regulating the integration of sensory information.Prog Brain Res. 1990;86:129-41. doi: 10.1016/s0079-6123(08)63172-6. Prog Brain Res. 1990. PMID: 1982365 Review.
-
Neuronal Circuits in Barrel Cortex for Whisker Sensory Perception.Physiol Rev. 2021 Jan 1;101(1):353-415. doi: 10.1152/physrev.00019.2019. Epub 2020 Aug 20. Physiol Rev. 2021. PMID: 32816652 Review.
Cited by
-
Whisker Vibrations and the Activity of Trigeminal Primary Afferents in Response to Airflow.J Neurosci. 2019 Jul 24;39(30):5881-5896. doi: 10.1523/JNEUROSCI.2971-18.2019. Epub 2019 May 16. J Neurosci. 2019. PMID: 31097620 Free PMC article.
-
Decoupling kinematics and mechanics reveals coding properties of trigeminal ganglion neurons in the rat vibrissal system.Elife. 2016 Jun 27;5:e13969. doi: 10.7554/eLife.13969. Elife. 2016. PMID: 27348221 Free PMC article.
-
Modeling forces and moments at the base of a rat vibrissa during noncontact whisking and whisking against an object.J Neurosci. 2014 Jul 23;34(30):9828-44. doi: 10.1523/JNEUROSCI.1707-12.2014. J Neurosci. 2014. PMID: 25057187 Free PMC article.
-
Demonstration of three-dimensional contact point determination and contour reconstruction during active whisking behavior of an awake rat.PLoS Comput Biol. 2022 Sep 15;18(9):e1007763. doi: 10.1371/journal.pcbi.1007763. eCollection 2022 Sep. PLoS Comput Biol. 2022. PMID: 36108064 Free PMC article.
-
Constraints on the deformation of the vibrissa within the follicle.PLoS Comput Biol. 2021 Apr 1;17(4):e1007887. doi: 10.1371/journal.pcbi.1007887. eCollection 2021 Apr. PLoS Comput Biol. 2021. PMID: 33793548 Free PMC article.
References
Publication types
MeSH terms
LinkOut - more resources
Full Text Sources
Research Materials