Time-series analysis for rapid event-related skin conductance responses
- PMID: 19686778
- PMCID: PMC2772899
- DOI: 10.1016/j.jneumeth.2009.08.005
Time-series analysis for rapid event-related skin conductance responses
Abstract
Event-related skin conductance responses (SCRs) are traditionally analysed by comparing the amplitude of individual peaks against a pre-stimulus baseline. Many experimental manipulations in cognitive neuroscience dictate paradigms with short inter trial intervals, precluding accurate baseline estimation for SCR measurements. Here, we present a novel and general approach to SCR analysis, derived from methods used in neuroimaging that estimate responses using a linear convolution model. In effect, the method obviates peak-scoring and makes use of the full SCR. We demonstrate, across three experiments, that the method has face validity in analysing reactions to a loud white noise and emotional pictures, can be generalised to paradigms where the shape of the response function is unknown and can account for parametric trial-by-trial effects. We suggest our approach provides greater flexibility in analysing SCRs than existing methods.
Figures
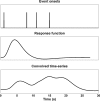
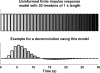
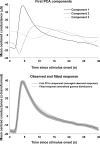
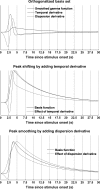
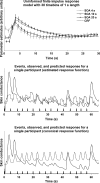
Similar articles
-
Separating individual skin conductance responses in a short interstimulus-interval paradigm.J Neurosci Methods. 2005 Jul 15;146(1):116-23. doi: 10.1016/j.jneumeth.2005.02.001. Epub 2005 Mar 3. J Neurosci Methods. 2005. PMID: 15935228
-
Development and validation of an unsupervised scoring system (Autonomate) for skin conductance response analysis.Int J Psychophysiol. 2014 Mar;91(3):186-93. doi: 10.1016/j.ijpsycho.2013.10.015. Epub 2013 Oct 31. Int J Psychophysiol. 2014. PMID: 24184342 Free PMC article.
-
Modelling event-related skin conductance responses.Int J Psychophysiol. 2010 Mar;75(3):349-56. doi: 10.1016/j.ijpsycho.2010.01.005. Epub 2010 Jan 20. Int J Psychophysiol. 2010. PMID: 20093150 Free PMC article.
-
Decomposition of skin conductance data by means of nonnegative deconvolution.Psychophysiology. 2010 Jul 1;47(4):647-58. doi: 10.1111/j.1469-8986.2009.00972.x. Epub 2010 Mar 5. Psychophysiology. 2010. PMID: 20230512 Free PMC article.
-
Dynamic causal modelling of anticipatory skin conductance responses.Biol Psychol. 2010 Sep;85(1):163-70. doi: 10.1016/j.biopsycho.2010.06.007. Epub 2010 Jun 25. Biol Psychol. 2010. PMID: 20599582 Free PMC article.
Cited by
-
Anxiety and the Neurobiology of Temporally Uncertain Threat Anticipation.J Neurosci. 2020 Oct 7;40(41):7949-7964. doi: 10.1523/JNEUROSCI.0704-20.2020. Epub 2020 Sep 21. J Neurosci. 2020. PMID: 32958570 Free PMC article.
-
Common Functional Brain States Encode both Perceived Emotion and the Psychophysiological Response to Affective Stimuli.Sci Rep. 2018 Oct 18;8(1):15444. doi: 10.1038/s41598-018-33621-6. Sci Rep. 2018. PMID: 30337576 Free PMC article.
-
Different brain networks mediate the effects of social and conditioned expectations on pain.Nat Commun. 2019 Sep 10;10(1):4096. doi: 10.1038/s41467-019-11934-y. Nat Commun. 2019. PMID: 31506426 Free PMC article.
-
A regret-induced status quo bias.J Neurosci. 2011 Mar 2;31(9):3320-7. doi: 10.1523/JNEUROSCI.5615-10.2011. J Neurosci. 2011. PMID: 21368043 Free PMC article.
-
Amygdala and prefrontal cortex activity varies with individual differences in the emotional response to psychosocial stress.Behav Neurosci. 2019 Apr;133(2):203-211. doi: 10.1037/bne0000305. Behav Neurosci. 2019. PMID: 30907618 Free PMC article.
References
-
- Alexander D.M., Trengove C., Johnston P., Cooper T., August J.P., Gordon E. Separating individual skin conductance responses in a short interstimulus-interval paradigm. J Neurosci Methods. 2005;146:116–123. - PubMed
-
- Amrhein C., Muhlberger A., Pauli P., Wiedemann G. Modulation of event-related brain potentials during affective picture processing: a complement to startle reflex and skin conductance response? Int J Psychophysiol. 2004;54:231–240. - PubMed
-
- Barry R.J., Feldmann S., Gordon E., Cocker K.I., Rennie C. Elicitation and habituation of the electrodermal orienting response in a short interstimulus interval paradigm. Int J Psychophysiol. 1993;15:247–253. - PubMed
-
- Boucsein W. Springer; Berlin: 1992. Electrodermal activity.
-
- Friston K.J., Ashburner J.T., Kiebel S.J., Nichols T.E., Penny W.D. Academic Press; London: 2008. Statistical parametric mapping: the analysis of functional brain images.
Publication types
MeSH terms
Grants and funding
LinkOut - more resources
Full Text Sources
Other Literature Sources