Do pseudo-absence selection strategies influence species distribution models and their predictions? An information-theoretic approach based on simulated data
- PMID: 19393082
- PMCID: PMC2680809
- DOI: 10.1186/1472-6785-9-8
Do pseudo-absence selection strategies influence species distribution models and their predictions? An information-theoretic approach based on simulated data
Abstract
Background: Multiple logistic regression is precluded from many practical applications in ecology that aim to predict the geographic distributions of species because it requires absence data, which are rarely available or are unreliable. In order to use multiple logistic regression, many studies have simulated "pseudo-absences" through a number of strategies, but it is unknown how the choice of strategy influences models and their geographic predictions of species. In this paper we evaluate the effect of several prevailing pseudo-absence strategies on the predictions of the geographic distribution of a virtual species whose "true" distribution and relationship to three environmental predictors was predefined. We evaluated the effect of using a) real absences b) pseudo-absences selected randomly from the background and c) two-step approaches: pseudo-absences selected from low suitability areas predicted by either Ecological Niche Factor Analysis: (ENFA) or BIOCLIM. We compared how the choice of pseudo-absence strategy affected model fit, predictive power, and information-theoretic model selection results.
Results: Models built with true absences had the best predictive power, best discriminatory power, and the "true" model (the one that contained the correct predictors) was supported by the data according to AIC, as expected. Models based on random pseudo-absences had among the lowest fit, but yielded the second highest AUC value (0.97), and the "true" model was also supported by the data. Models based on two-step approaches had intermediate fit, the lowest predictive power, and the "true" model was not supported by the data.
Conclusion: If ecologists wish to build parsimonious GLM models that will allow them to make robust predictions, a reasonable approach is to use a large number of randomly selected pseudo-absences, and perform model selection based on an information theoretic approach. However, the resulting models can be expected to have limited fit.
Figures
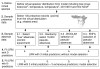
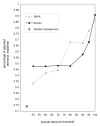
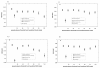
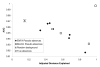
Similar articles
-
Where did they not go? Considerations for generating pseudo-absences for telemetry-based habitat models.Mov Ecol. 2021 Feb 17;9(1):5. doi: 10.1186/s40462-021-00240-2. Mov Ecol. 2021. PMID: 33596991 Free PMC article.
-
On the importance of predictor choice, modelling technique, and number of pseudo-absences for bioclimatic envelope model performance.Ecol Evol. 2020 Oct 16;10(21):12307-12317. doi: 10.1002/ece3.6859. eCollection 2020 Nov. Ecol Evol. 2020. PMID: 33209289 Free PMC article.
-
Observer-oriented approach improves species distribution models from citizen science data.Ecol Evol. 2020 Sep 26;10(21):12104-12114. doi: 10.1002/ece3.6832. eCollection 2020 Nov. Ecol Evol. 2020. PMID: 33209273 Free PMC article.
-
Distribution models for koalas in South Australia using citizen science-collected data.Ecol Evol. 2014 Jun;4(11):2103-14. doi: 10.1002/ece3.1094. Epub 2014 Apr 28. Ecol Evol. 2014. PMID: 25360252 Free PMC article. Review.
-
Applications of Monte Carlo Simulation in Modelling of Biochemical Processes.In: Mode CJ, editor. Applications of Monte Carlo Methods in Biology, Medicine and Other Fields of Science [Internet]. Rijeka (HR): InTech; 2011 Feb 28. Chapter 4. In: Mode CJ, editor. Applications of Monte Carlo Methods in Biology, Medicine and Other Fields of Science [Internet]. Rijeka (HR): InTech; 2011 Feb 28. Chapter 4. PMID: 28045483 Free Books & Documents. Review.
Cited by
-
Using pseudo-absence models to test for environmental selection in marine movement ecology: the importance of sample size and selection strength.Mov Ecol. 2022 Dec 29;10(1):60. doi: 10.1186/s40462-022-00362-1. Mov Ecol. 2022. PMID: 36581885 Free PMC article.
-
The potential impact of invasive woody oil plants on protected areas in China under future climate conditions.Sci Rep. 2018 Jan 18;8(1):1041. doi: 10.1038/s41598-018-19477-w. Sci Rep. 2018. PMID: 29348468 Free PMC article.
-
Topographic models for predicting malaria vector breeding habitats: potential tools for vector control managers.Parasit Vectors. 2013 Jan 16;6:14. doi: 10.1186/1756-3305-6-14. Parasit Vectors. 2013. PMID: 23324389 Free PMC article.
-
The effects of sampling bias and model complexity on the predictive performance of MaxEnt species distribution models.PLoS One. 2013;8(2):e55158. doi: 10.1371/journal.pone.0055158. Epub 2013 Feb 14. PLoS One. 2013. PMID: 23457462 Free PMC article.
-
Estimating hantavirus risk in southern Argentina: a GIS-based approach combining human cases and host distribution.Viruses. 2014 Jan 14;6(1):201-22. doi: 10.3390/v6010201. Viruses. 2014. PMID: 24424500 Free PMC article.
References
-
- Leathwick JR. Are New Zealand's Nothofagus species in equilibrium with their environment? Journal of Vegetation Science. 1998;9:719–732. doi: 10.2307/3237290. - DOI
-
- Wisz MS, Walther BA, Rahbek C. Using potential distributions to explore determinants of Western Palaearctic migratory songbird species richness in sub-Saharan Africa. Journal of Biogeography. 2007;34:828–841. doi: 10.1111/j.1365-2699.2006.01661.x. - DOI
-
- Robertson MP, Caithness N, Villet MH. A PCA-based modelling technique for predicting environmental suitability for organisms from presence records. Diversity and Distributions. 2001;7:15–27. doi: 10.1046/j.1472-4642.2001.00094.x. - DOI
-
- Busby JR. BIOCLIM – a bioclimate analysis and prediction system. In: Margules CR, Austin MP, editor. Nature Conservation: Cost Effective Biological Surveys and Data Analysis. Canberra, Australia: CSIRO; 1991. pp. 64–68.
MeSH terms
LinkOut - more resources
Full Text Sources