SUN: A Bayesian framework for saliency using natural statistics
- PMID: 19146264
- PMCID: PMC7360059
- DOI: 10.1167/8.7.32
SUN: A Bayesian framework for saliency using natural statistics
Abstract
We propose a definition of saliency by considering what the visual system is trying to optimize when directing attention. The resulting model is a Bayesian framework from which bottom-up saliency emerges naturally as the self-information of visual features, and overall saliency (incorporating top-down information with bottom-up saliency) emerges as the pointwise mutual information between the features and the target when searching for a target. An implementation of our framework demonstrates that our model's bottom-up saliency maps perform as well as or better than existing algorithms in predicting people's fixations in free viewing. Unlike existing saliency measures, which depend on the statistics of the particular image being viewed, our measure of saliency is derived from natural image statistics, obtained in advance from a collection of natural images. For this reason, we call our model SUN (Saliency Using Natural statistics). A measure of saliency based on natural image statistics, rather than based on a single test image, provides a straightforward explanation for many search asymmetries observed in humans; the statistics of a single test image lead to predictions that are not consistent with these asymmetries. In our model, saliency is computed locally, which is consistent with the neuroanatomy of the early visual system and results in an efficient algorithm with few free parameters.
Figures
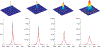
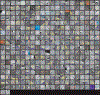
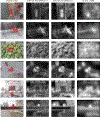
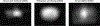
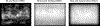
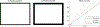
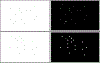
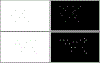

Similar articles
-
What stands out in a scene? A study of human explicit saliency judgment.Vision Res. 2013 Oct 18;91:62-77. doi: 10.1016/j.visres.2013.07.016. Epub 2013 Aug 15. Vision Res. 2013. PMID: 23954536
-
What do saliency models predict?J Vis. 2014 Mar 11;14(3):14. doi: 10.1167/14.3.14. J Vis. 2014. PMID: 24618107 Free PMC article.
-
A unified computational framework for visual attention dynamics.Prog Brain Res. 2019;249:183-188. doi: 10.1016/bs.pbr.2019.01.001. Epub 2019 Feb 8. Prog Brain Res. 2019. PMID: 31325977
-
Probabilistic Computations for Attention, Eye Movements, and Search.Annu Rev Vis Sci. 2017 Sep 15;3:319-342. doi: 10.1146/annurev-vision-102016-061220. Epub 2017 Jul 26. Annu Rev Vis Sci. 2017. PMID: 28746814 Review.
-
On the plausibility of the discriminant center-surround hypothesis for visual saliency.J Vis. 2008 Jun 13;8(7):13.1-18. doi: 10.1167/8.7.13. J Vis. 2008. PMID: 19146246 Review.
Cited by
-
Saliency models perform best for women's and young adults' fixations.Commun Psychol. 2023 Nov 17;1(1):34. doi: 10.1038/s44271-023-00035-8. Commun Psychol. 2023. PMID: 39242730 Free PMC article.
-
A dual foveal-peripheral visual processing model implements efficient saccade selection.J Vis. 2020 Aug 3;20(8):22. doi: 10.1167/jov.20.8.22. J Vis. 2020. PMID: 38755789 Free PMC article.
-
An improved saliency model of visual attention dependent on image content.Front Hum Neurosci. 2023 Feb 28;16:862588. doi: 10.3389/fnhum.2022.862588. eCollection 2022. Front Hum Neurosci. 2023. PMID: 36926377 Free PMC article.
-
Five Factors that Guide Attention in Visual Search.Nat Hum Behav. 2017 Mar;1(3):0058. doi: 10.1038/s41562-017-0058. Epub 2017 Mar 8. Nat Hum Behav. 2017. PMID: 36711068 Free PMC article.
-
Multiscale Cascaded Attention Network for Saliency Detection Based on ResNet.Sensors (Basel). 2022 Dec 16;22(24):9950. doi: 10.3390/s22249950. Sensors (Basel). 2022. PMID: 36560319 Free PMC article.
References
-
- Barlow H (1994). What is the computational goal of the neocortex? In Koch C (Ed.), Large scale neuronal theories of the brain (pp. 1–22). Cambridge, MA: MIT Press.
-
- Bruce N, & Tsotsos J (2006). Saliency based on information maximization In Weiss Y, Schölkopf B, & Platt J (Eds.), Advances in neural information processing systems 18 (pp. 155–162). Cambridge, MA: MIT Press.
-
- Bundesen C (1990). A theory of visual attention. Psychological Review, 97, 523–547. - PubMed
-
- Carmi R, & Itti L (2006). The role of memory in guiding attention during natural vision. Journal of Vision, 6(9):4, 898–914, http://journalofvision.org/6/9/4/, doi:10.1167/6.9.4. [Article] - DOI - PubMed
Publication types
MeSH terms
Grants and funding
LinkOut - more resources
Full Text Sources
Other Literature Sources
Miscellaneous