Gene selection and classification of microarray data using random forest
- PMID: 16398926
- PMCID: PMC1363357
- DOI: 10.1186/1471-2105-7-3
Gene selection and classification of microarray data using random forest
Abstract
Background: Selection of relevant genes for sample classification is a common task in most gene expression studies, where researchers try to identify the smallest possible set of genes that can still achieve good predictive performance (for instance, for future use with diagnostic purposes in clinical practice). Many gene selection approaches use univariate (gene-by-gene) rankings of gene relevance and arbitrary thresholds to select the number of genes, can only be applied to two-class problems, and use gene selection ranking criteria unrelated to the classification algorithm. In contrast, random forest is a classification algorithm well suited for microarray data: it shows excellent performance even when most predictive variables are noise, can be used when the number of variables is much larger than the number of observations and in problems involving more than two classes, and returns measures of variable importance. Thus, it is important to understand the performance of random forest with microarray data and its possible use for gene selection.
Results: We investigate the use of random forest for classification of microarray data (including multi-class problems) and propose a new method of gene selection in classification problems based on random forest. Using simulated and nine microarray data sets we show that random forest has comparable performance to other classification methods, including DLDA, KNN, and SVM, and that the new gene selection procedure yields very small sets of genes (often smaller than alternative methods) while preserving predictive accuracy.
Conclusion: Because of its performance and features, random forest and gene selection using random forest should probably become part of the "standard tool-box" of methods for class prediction and gene selection with microarray data.
Figures
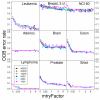
Similar articles
-
An introspective comparison of random forest-based classifiers for the analysis of cluster-correlated data by way of RF++.PLoS One. 2009 Sep 18;4(9):e7087. doi: 10.1371/journal.pone.0007087. PLoS One. 2009. PMID: 19763254 Free PMC article.
-
Tumor classification ranking from microarray data.BMC Genomics. 2008 Sep 16;9 Suppl 2(Suppl 2):S21. doi: 10.1186/1471-2164-9-S2-S21. BMC Genomics. 2008. PMID: 18831787 Free PMC article.
-
Identification of differential gene expression for microarray data using recursive random forest.Chin Med J (Engl). 2008 Dec 20;121(24):2492-6. Chin Med J (Engl). 2008. PMID: 19187584
-
Unsupervised pattern recognition: an introduction to the whys and wherefores of clustering microarray data.Brief Bioinform. 2005 Dec;6(4):331-43. doi: 10.1093/bib/6.4.331. Brief Bioinform. 2005. PMID: 16420732 Review.
-
Optimal features selection in the high dimensional data based on robust technique: Application to different health database.Heliyon. 2024 Sep 2;10(17):e37241. doi: 10.1016/j.heliyon.2024.e37241. eCollection 2024 Sep 15. Heliyon. 2024. PMID: 39296019 Free PMC article. Review.
Cited by
-
Functional knowledge transfer for high-accuracy prediction of under-studied biological processes.PLoS Comput Biol. 2013;9(3):e1002957. doi: 10.1371/journal.pcbi.1002957. Epub 2013 Mar 14. PLoS Comput Biol. 2013. PMID: 23516347 Free PMC article.
-
Transcriptome classification reveals molecular subtypes in psoriasis.BMC Genomics. 2012 Sep 12;13:472. doi: 10.1186/1471-2164-13-472. BMC Genomics. 2012. PMID: 22971201 Free PMC article.
-
Robust edge-based biomarker discovery improves prediction of breast cancer metastasis.BMC Bioinformatics. 2020 Sep 30;21(Suppl 14):359. doi: 10.1186/s12859-020-03692-2. BMC Bioinformatics. 2020. PMID: 32998692 Free PMC article.
-
Random Forests for Global and Regional Crop Yield Predictions.PLoS One. 2016 Jun 3;11(6):e0156571. doi: 10.1371/journal.pone.0156571. eCollection 2016. PLoS One. 2016. PMID: 27257967 Free PMC article.
-
Automated identification of protein-ligand interaction features using Inductive Logic Programming: a hexose binding case study.BMC Bioinformatics. 2012 Jul 11;13:162. doi: 10.1186/1471-2105-13-162. BMC Bioinformatics. 2012. PMID: 22783946 Free PMC article.
References
-
- Lee JW, Lee JB, Park M, Song SH. An extensive evaluation of recent classification tools applied to microarray data. Computation Statistics and Data Analysis. 2005;48:869–885.
-
- Yeung KY, Bumgarner RE, Raftery AE. Bayesian model averaging: development of an improved multi-class, gene selection and classification tool for microarray data. Bioinformatics. 2005;21:2394–2402. - PubMed
-
- Hua J, Xiong Z, Lowey J, Suh E, Dougherty ER. Optimal number of features as a function of sample size for various classification rules. Bioinformatics. 2005;21:1509–1515. - PubMed
-
- Li Y, Campbell C, Tipping M. Bayesian automatic relevance determination algorithms for classifying gene expression data. Bioinformatics. 2002;18:1332–1339. - PubMed
Publication types
MeSH terms
LinkOut - more resources
Full Text Sources
Other Literature Sources