Usually in this space I like to share interesting things that I find
out; you might call it a research-epistle-publish loop. Today, though,
I come not with answers, but with questions, or rather one question, but
with fractal surface area: what is the value proposition of
generational garbage collection?
hypothesis
The conventional wisdom is encapsulated in a 2004 Blackburn, Cheng, and
McKinley paper, “Myths and Realities: The Performance Impact of
Garbage
Collection”,
which compares whole-heap mark-sweep and copying collectors to their
generational counterparts, using the Jikes RVM as a test harness. (It
also examines a generational reference-counting collector, which is an
interesting predecessor to the 2022
LXR work
by Zhao, Blackburn, and McKinley.)
The paper finds that generational collectors spend less time than their
whole-heap counterparts for a given task. This is mainly due to less
time spent collecting, because generational collectors avoid
tracing/copying work for older objects that mostly stay in the same
place in the live object graph.
The paper also notes an improvement for mutator time under generational
GC, but only for the generational mark-sweep collector, which it
attributes to the locality and allocation speed benefit of bump-pointer
allocation in the nursery. However for copying collectors, generational
GC tends to slow down the mutator, probably because of the write
barrier, but in the end lower collector times still led to lower total
times.
So, I expected generational collectors to always exhibit lower
wall-clock times than whole-heap collectors.
test workbench
In whippet, I have a garbage
collector with an abstract API that specializes at compile-time to the
mutator’s object and root-set representation and to the collector’s
allocator, write barrier, and other interfaces. I embed it in
whiffle, a simple Scheme-to-C
compiler that can run some small multi-threaded benchmarks, for example
the classic Gabriel benchmarks. We can then test those benchmarks
against different collectors, mutator (thread) counts, and heap sizes.
I expect that the generational parallel copying collector takes less
time than the whole-heap parallel copying collector.
results?
So, I ran some benchmarks. Take the splay-tree benchmark, derived from
Octane’s
splay.js. I
have a port to Scheme, and the results are... not good!
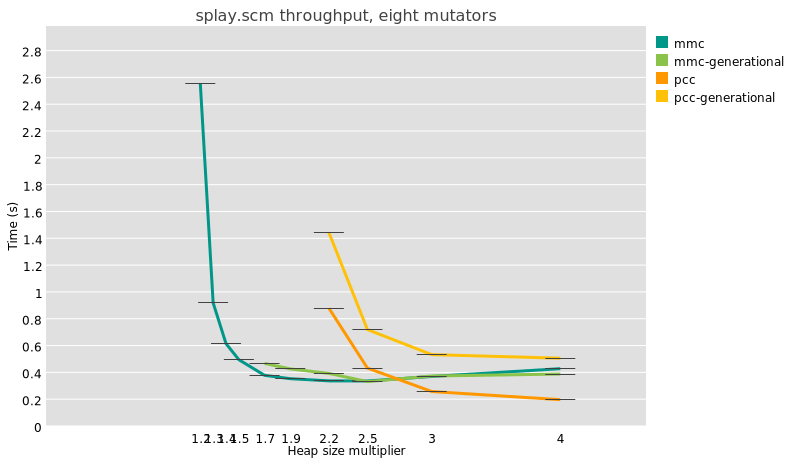
In this graph the “pcc” series is the whole-heap copying collector, and
“generational-pcc” is the generational counterpart, with a nursery sized
such that after each collection, its size is 2 MB times the number of
active mutator threads in the last collector. So, for this test with
eight threads, on my 8-core Ryzen 7 7840U laptop, the nursery is 16MB
including the copy reserve, which happens to be the same size as the L3
on this CPU. New objects are kept in the nursery one cycle before being
promoted to the old generation.
There are also results for “mmc” and “generational-mmc”
collectors,
which use an Immix-derived algorithm that allows for bump-pointer
allocation but which doesn’t require a copy reserve. There, the
generational collectors use a sticky mark-bit
algorithm,
which has very different performance characteristics as promotion is
in-place, and the nursery is as large as the available heap size.
The salient point is that at all heap sizes, and for these two very
different configurations (mmc and pcc), generational collection takes
more time than whole-heap collection. It’s not just the splay benchmark
either; I see the same thing for the very different nboyer
benchmark.
What is the deal?
I am honestly quite perplexed by this state of affairs. I wish I had a
narrative to tie this together, but in lieu of that, voici some
propositions and observations.
“generational collection is good because bump-pointer allocation”
Sometimes people say that the reason generational collection is good is
because you get bump-pointer allocation, which has better locality and
allocation speed. This is misattribution: it’s bump-pointer allocators
that have these benefits. You can have them in whole-heap copying
collectors, or you can have them in whole-heap mark-compact or immix
collectors that bump-pointer allocate into the holes. Or, true, you can
have them in generational collectors with a copying nursery but a
freelist-based mark-sweep allocator. But also you can have generational
collectors without bump-pointer allocation, for free-list
sticky-mark-bit collectors. To simplify this panorama to “generational
collectors have good allocators” is incorrect.
“generational collection lowers pause times”
It’s true, generational GC does lower median pause times:
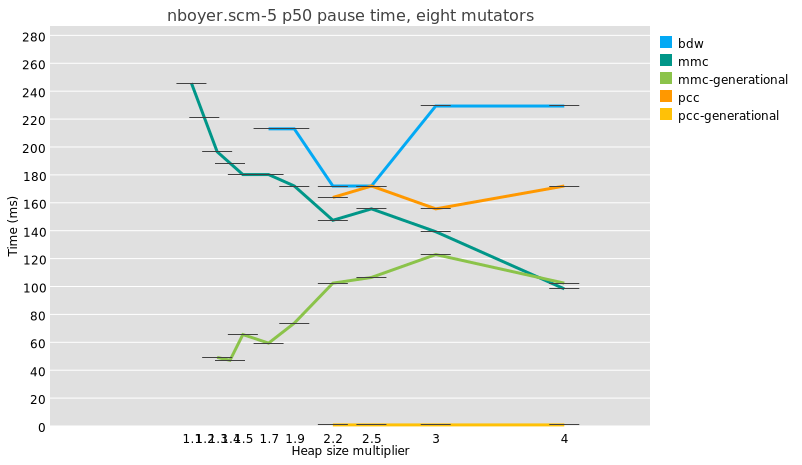
But because a major collection is usually slightly more work under
generational GC than in a whole-heap system, because of e.g. the need to
reset remembered sets, the maximum pauses are just as big and even a
little bigger:
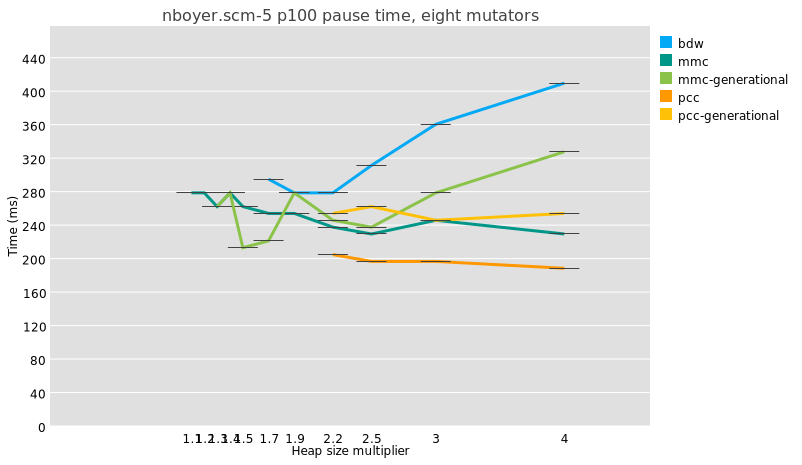
I am not even sure that it is meaningful to compare median pause times
between generational and non-generational collectors, given that the
former perform possibly orders of magnitude more collections than the
latter.
Doing fewer whole-heap traces is good, though, and in the ideal case,
the less frequent major traces under generational collectors allows time
for concurrent tracing, which is the true mitigation for long pause
times.
is it whiffle?
Could it be that the test harness I am using is in some way
unrepresentative? I don’t have more than one test harness for Whippet
yet. I will start work on a second Whippet embedder within the next few
weeks, so perhaps we will have an answer there. Still, there is ample
time spent in GC pauses in these benchmarks, so surely as a GC workload
Whiffle has some utility.
One reasons that Whiffle might be unrepresentative is that it is an
ahead-of-time compiler, whereas nursery addresses are assigned at
run-time. Whippet exposes the necessary information to allow a
just-in-time compiler to specialize write barriers, for example the
inline check that the field being mutated is not in the nursery, and an
AOT compiler can’t encode this as an immediate. But it seems a small
detail.
Also, Whiffle doesn’t do much compiler-side work to elide write
barriers. Could the cost of write barriers be over-represented in
Whiffle, relative to a production language run-time?
Relatedly, Whiffle is just a baseline compiler. It does some partial
evaluation but no CFG-level optimization, no contification, no nice
closure conversion, no specialization, and so on: is it not
representative because it is not an optimizing compiler?
is it something about the nursery size?
How big should the nursery be? I have no idea.
As a thought experiment, consider the case of a 1 kilobyte nursery. It
is probably too small to allow the time for objects to die young, so the
survival rate at each minor collection would be high. Above a certain
survival rate, generational GC is probably a lose, because your program
violates the weak generational hypothesis: it introduces a needless copy
for all survivors, and a synchronization for each minor GC.
On the other hand, a 1 GB nursery is probably not great either. It is
plenty large enough to allow objects to die young, but the number of
survivor objects in a space that large is such that pause times would
not be very low, which is one of the things you would like in
generational GC. Also, you lose out on locality: a significant fraction
of the objects you traverse are probably out of cache and might even
incur TLB misses.
So there is probably a happy medium somewhere. My instinct is that for
a copying nursery, you want to make it about as big as L3 cache, which
on my 8-core laptop is 16 megabytes. Systems are different sizes
though; in Whippet my current heuristic is to reserve 2 MB of nursery
per core that was active in the previous cycle, so if only 4 threads are
allocating, you would have a 8 MB nursery. Is this good? I don’t know.
is it something about the benchmarks?
I don’t have a very large set of benchmarks that run on Whiffle, and
they might not be representative. I mean, they are microbenchmarks.
One question I had was about heap sizes. If a benchmark’s maximum heap
size fits in L3, which is the case for some of them, then probably
generational GC is a wash, because whole-heap collection stays in cache.
When I am looking at benchmarks that evaluate generational GC, I make
sure to choose those that exceed L3 size by a good factor, for example
the 8-mutator splay benchmark in which minimum heap size peaks at 300
MB, or the 8-mutator nboyer-5 which peaks at 1.6 GB.
But then, should nursery size scale with total heap size? I don’t know!
Incidentally, the way that I scale these benchmarks to multiple mutators
is a bit odd: they are serial benchmarks, and I just run some number of
threads at a time, and scale the heap size accordingly, assuming that
the minimum size when there are 4 threads is four times the minimum size
when there is just one thread. However, multithreaded programs are
unreliable,
in the sense that there is no heap size under which they fail and above
which they succeed; I quote:
"Consider 10 threads each of which has a local object graph that is
usually 10 MB but briefly 100MB when calculating: usually when GC
happens, total live object size is 10×10MB=100MB, but sometimes as
much as 1 GB; there is a minimum heap size for which the program
sometimes works, but also a minimum heap size at which it always
works."
is it the write barrier?
A generational collector partitions objects into old and new sets, and a
minor collection starts by visiting all old-to-new edges, called the
“remembered set”. As the program runs, mutations to old objects might
introduce new old-to-new edges. To maintain the remembered set in a
generational collector, the mutator invokes write barriers: little
bits of code that run when you mutate a field in an object. This is
overhead relative to non-generational configurations, where the mutator
doesn’t have to invoke collector code when it sets fields.
So, could it be that Whippet’s write barriers or remembered set are
somehow so inefficient that my tests are unrepresentative of the state
of the art?
I used to use card-marking barriers, but I started to suspect they cause
too much overhead during minor GC and introduced too much cache
contention. I switched to precise field-logging
barriers
some months back for Whippet’s Immix-derived space, and we use the same
kind of barrier in the generational copying (pcc) collector. I think
this is state of the art. I need to see if I can find a configuration
that allows me to measure the overhead of these barriers, independently
of other components of a generational collector.
is it something about the generational mechanism?
A few months ago, my only generational collector used the sticky
mark-bit
algorithm, which is an unconventional configuration: its nursery is not
contiguous, non-moving, and can be as large as the heap. This is part
of the reason that I implemented generational support for the parallel
copying collector, to have a different and more conventional collector
to compare against. But generational collection loses on some of these
benchmarks in both places!
is it something about collecting more often?
On one benchmark which repeatedly constructs some trees and then
verifies them, I was seeing terrible results for generational GC, which
I realized were because of cooperative safepoints: generational GC
collects more often, so it requires that all threads reach safepoints
more often, and the non-allocating verification phase wasn’t emitting
any safepoints. I had to change the compiler to emit safepoints at
regular intervals (in my case, on function entry), and it sped up the
generational collector by a significant amount.
This is one instance of a general observation, which is that any work
that doesn’t depend on survivor size in a GC pause is more expensive
with a generational collector, which runs more collections.
Synchronization can be a cost. I had one bug in which tracing
ephemerons did work proportional to the size of the whole heap, instead
of the nursery; I had to specifically add generational support for the
way Whippet deals with ephemerons during a collection to reduce this
cost.
is it something about collection frequency?
Looking deeper at the data, I have partial answers for the splay benchmark,
and they are annoying :)
Splay doesn’t actually allocate all that much garbage. At a 2.5x heap,
the stock parallel MMC collector (in-place, sticky mark bit)
collects... one time. That’s all. Same for the generational MMC
collector, because the first collection is always major. So at 2.5x we
would expect the generational collector to be slightly slower. The
benchmark is simply not very good – or perhaps the most generous
interpretation is that it represents tasks that allocate 40 MB or so of
long-lived data and not much garbage on top.
Also at 2.5x heap, the whole-heap copying collector runs 9 times, and
the generational copying collector does 293 minor collections and... 9
major collections. We are not reducing the number of major GCs. It
means either the nursery is too small, so objects aren’t dying young
when they could, or the benchmark itself doesn’t conform to the weak
generational hypothesis.
At a 1.5x heap, the copying collector doesn’t have enough space to run.
For MMC, the non-generational variant collects 7 times, and generational
MMC times out. Timing out indicates a bug, I think. Annoying!
I tend to think that if I get results and there were fewer than, like, 5
major collections for a whole-heap collector, that indicates that the
benchmark is probably inapplicable at that heap size, and I should
somehow surface these anomalies in my analysis scripts.
collecting more often redux
Doing a similar exercise for nboyer at 2.5x heap with 8 threads (4GB for
1.6GB live data), I see that pcc did 20 major collections, whereas
generational pcc lowered that to 8 major collections and 3471 minor
collections. Could it be that there are still too many fixed costs
associated with synchronizing for global stop-the-world minor
collections? I am going to have to add some fine-grained tracing to
find out.
conclusion?
I just don’t know! I want to believe that generational collection was an out-and-out win,
but I haven’t yet been able to prove it is true.
I do have some homework to do. I need to find a way to test the
overhead of my write barrier – probably using the MMC collector and
making it only do major collections. I need to fix generational-mmc for
splay and a 1.5x heap. And I need to do some fine-grained performance
analysis for minor collections in large heaps.
Enough for today. Feedback / reactions very welcome. Thanks for
reading and happy hacking!